Introducing the 2024 AstroAI Workshop, June 17-21!
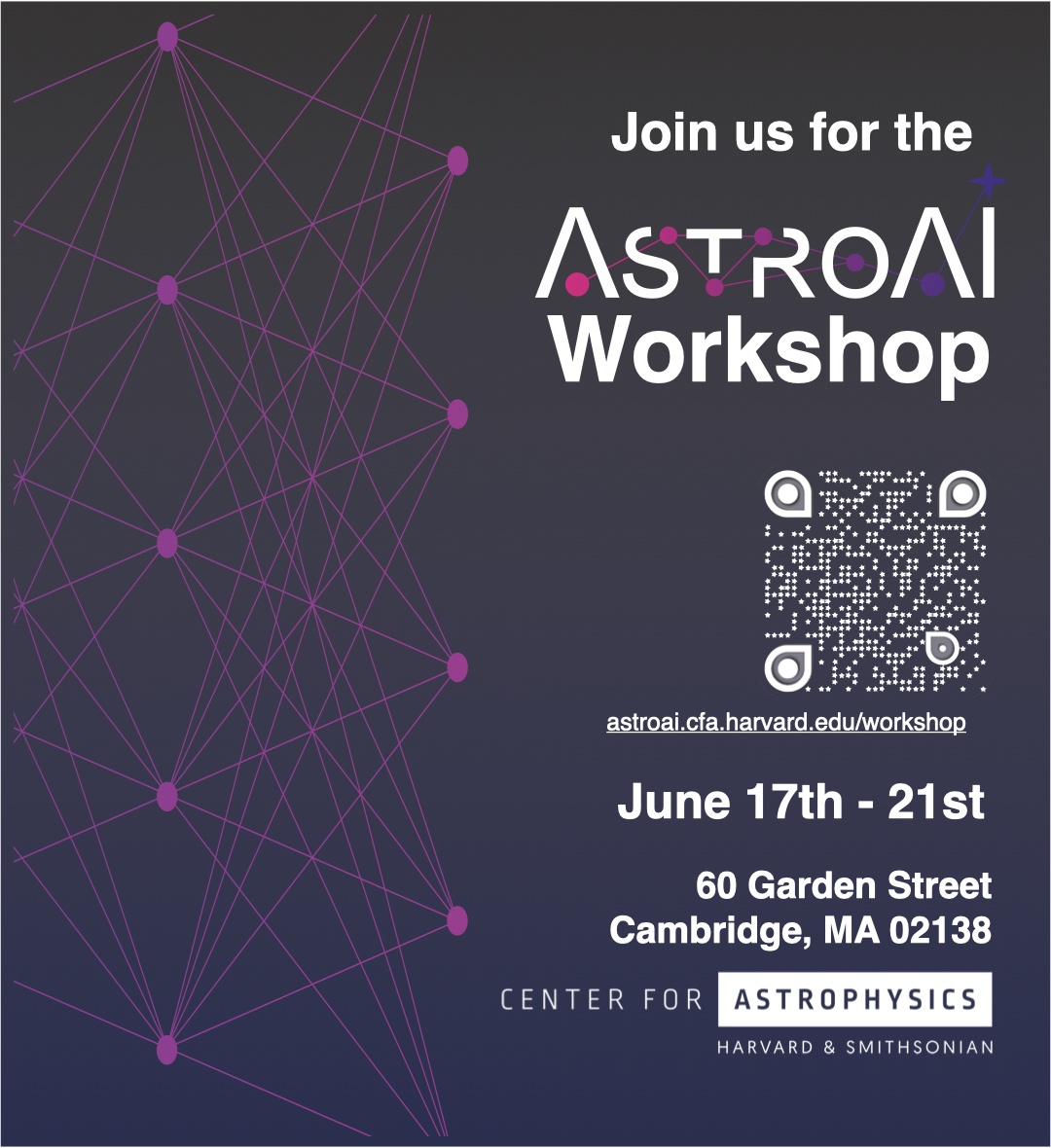
Check here for more information.
-
AstroAI is at SXSW 2024
Revolutionizing Astrophysics with AI
Tuesday, March 12, 2024 from 11:30 AM - 12:30 PM, CT in Salon C of the Hilton Austin Downtown
If you are at SXSW 2024, come visit our session: https://schedule.sxsw.com/2024/events/PP1145780
Read More... -
Are You Interested in Working With AstroAI?
AstroAI is actively looking for collaborators and researchers at all career stages. If you are interested in working with AstroAI, please check out our Apply page for more details.
Read More... -
Camilo Fosco in the News!
AstroAI member Camilo Fosco was recently interviewed by Wired! The interview discusses how people and AI view artwork as memorable.
Read More... -
Congratulations to Mayeul Aubin
Huge congrats to AstroAI’s first intern, Mayeul Aubin, and our dedicated team for clinching the #1 spot in the Ariel Data Challenge by the European Space Agency! Swift models, groundbreaking domain knowledge, and incredible team effort powered our work. Proving once again that the AstroAI model shines bright in the universe of machine learning!
Read More... -
Thaddaeus Kiker Finishes 10th in the 2023 Regeneron Science Talent Search
AstroAI congratulates Thaddaeus Kiker finishing 10th in the 2023 Regeneron Science Talent Search.
Read More... -
AstroAI Member Carolina Cuesta-Lazaro wins Botton Foundation Fellowship
AstroAI affiliate Carolina Cuesta-Lazaro was one of three MIT postdocs to be awarded the inagural Mauricio and Carlota Botton Foundation fellowship.
Read More... -
Javier Viaña Pérez Gives a TEDx Talk about Interpretable AI
AstroAI affiliate Javier Viaña Pérez recently gave a talk at TEDxBoston discussing the importance of explainability and interpretability in the AI community.
Read More... -
Floor Broekgaarden Gives a 2023 Harvard Horizons Scholars Talk
On April 11, 2023 in Sanders Theatre, 2023 Harvard Horizons Scholar, Harvard Griffin GSAS astronomy student, and AstroAI leadership member Floor Broekgaarden presented her PhD research in her talk “Gravitational Wave Paleontology: a new Frontier to Explore the Lives of Stars to the Edge of our Observable Universe.”
Read More... -
AstroAI Publication Investigating the Background Dynamics of the Universe
The field of machine learning has drawn increasing interest from various other fields due to the success of its methods at solving a plethora of different problems. An application of these has been to train artificial neural networks to solve differential equations without the need of a numerical solver. This particular application offers an alternative to conventional numerical methods, with advantages such as lower memory required to store solutions, parallelization, and, in some cases, a lower overall computational cost than its numerical counterparts. In this work, we train artificial neural networks to represent a bundle of solutions of the differential equations that govern the background dynamics of the Universe for four different models. The models we have chosen are Λ CDM , the Chevallier-Polarski-Linder parametric dark energy model, a quintessence model with an exponential potential, and the Hu-Sawicki f (R ) model. We use the solutions that the networks provide to perform statistical analyses to estimate the values of each model’s parameters with observational data; namely, estimates of the Hubble parameter from cosmic chronometers, type Ia supernovae data from the Pantheon compilation, and measurements from baryon acousstic oscillations. The results we obtain for all models match similar estimations done in the literature using numerical solvers. In addition, we estimate the error of the solutions that the trained networks provide by comparing them with the analytical solution when there is one, or to a high-precision numerical solution when there is not. Through these estimations we find that the error of the solutions is at most ∼1 % in the region of the parameter space that concerns the 95% confidence regions that we find using the data, for all models and all statistical analyses performed in this work. Some of these results are made possible by improvements to the method of solving differential equations with artificial neural networks conceived in this work.
Read More... -
AstroAI Publication Mapping the Variability of a Binary Black Hole
Black hole X-ray binary systems (BHBs) contain a close companion star accreting onto a stellar-mass black hole. A typical BHB undergoes transient outbursts during which it exhibits a sequence of long-lived spectral states, each of which is relatively stable. GRS 1915+105 is a unique BHB that exhibits an unequaled number and variety of distinct variability patterns in X-rays. Many of these patterns contain unusual behaviour not seen in other sources. These variability patterns have been sorted into different classes based on count rate and color characteristics by Belloni et al (2000). In order to remove human decision-making from the pattern-recognition process, we employ an unsupervised machine learning algorithm called an auto-encoder to learn what classifications are naturally distinct by allowing the algorithm to cluster observations. We focus on observations taken by the Rossi X-ray Timing Explorer’s Proportional Counter Array. We find that the auto-encoder closely groups observations together that are classified as similar under the Belloni et al (2000) system, but that there is reasonable grounds for defining each class as made up of components from 3 groups of distinct behaviour.
Read More... -
Congratulations to Thaddaeus Kiker
AstroAI congratulates Thaddaeus Kiker for being one of the Regeneron Science Talent Search 2023 Finalists.
Read More... -
AstroAI Publication Examining TESS Lightcurves
With the upcoming plethora of astronomical time-domain datasets and surveys, anomaly detection as a way to discover new types of variable stars and transients has inspired a new wave of research. Yet, the fundamental definition of what constitutes an anomaly and how this depends on the overall properties of the population of light curves studied remains a discussed issue. Building on a previous study focused on Kepler light curves, we present an analysis that uses the Unsupervised Random Forest to search for anomalies in TESS light curves. We provide a catalogue of anomalous light curves, classify them according to their variability characteristics and associate their anomalous nature to any particular evolutionary stage or astrophysical configuration. For anomalies belonging to known classes (e.g. eclipsing binaries), we have investigated which physical parameters drive the anomaly score. We find a combination of unclassified anomalies and objects of a known class with outlying physical configurations, such as rapid pulsators, deep eclipsing binaries of long periods, and irregular light curves due to obscuration in YSOs. Remarkably, we find that the set of anomalous types differ between the Kepler and TESS datasets, indicating that the overall properties of the parent population are an important driver of anomalous behaviour.
Read More...